1
Overview
Konica Minolta has been actively promoting projects using AI. However, annotation during the development of AI models requires manually processing a large amount of data, which is a major challenge. To address this challenge, annotation tools, such as CVAT1) and LabelStudio,2) offer an automatic annotation function, but do not have a function to retrain AI models for labeling, making it difficult to improve accuracy and cope with different projects. QuickAnno developed by Konica Minolta features an automatic annotation function for the dedicated AI model (hereafter referred to as “Anno-AI model”) and a retraining function using data in the tool. In addition, it avoids having to construct Anno-AI models from scratch and achieves efficient automatic annotation by enabling centralized management and sharing of multiple Anno-AI models and by performing additional training based on models having similar requirements. With these functions, this system improves the efficiency of AI development.
2
Details
■Configuration
QuickAnno is a system that realizes automatic annotation depending on data characteristics and requirements by using Anno-AI. It consists of annotation tools, such as CVAT and Label Studio, and MLflow,3) which is a tool for managing training results. To increase the efficiency of linkage between the tools, Konica Minolta develops and implements integrated UI and Anno-AI operation tools. Users can automatically annotate data, check and manage the training results, and retrain Anno-AI on a single GUI (Fig. 1).
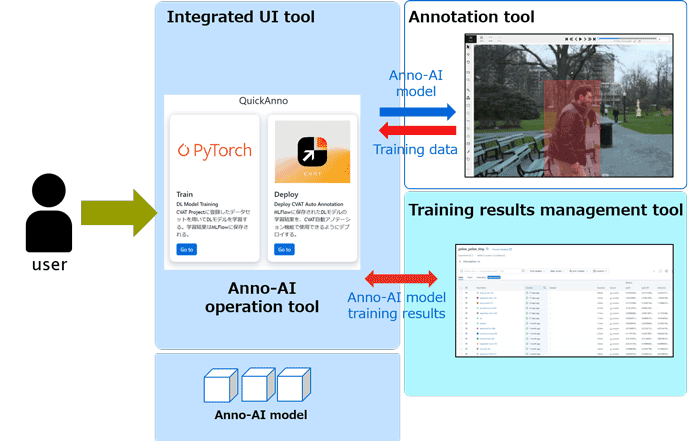
Fig. 1 Architecture of QuickAnno
■Functions / Features / Applications
In this system, Anno-AI performs annotation of the target data, and users check and correct the results. This significantly reduces the work time compared to manual work from scratch. The training data created by automatic annotation and correction by users is stored in this system and used for training AI models for projects. The data corrected by users is also used for retraining to improve the automatic annotation accuracy of Anno-AI. Conventional annotation tools required an external environment to retrain AI models for automatic annotation. However, this system can directly select the correction data in storage and perform retraining. This function optimizes the “automatic annotation by Anno-AI → correction → retraining” cycle, enabling the continuous improvement and utilization of Anno-AI for each project (Fig. 2).
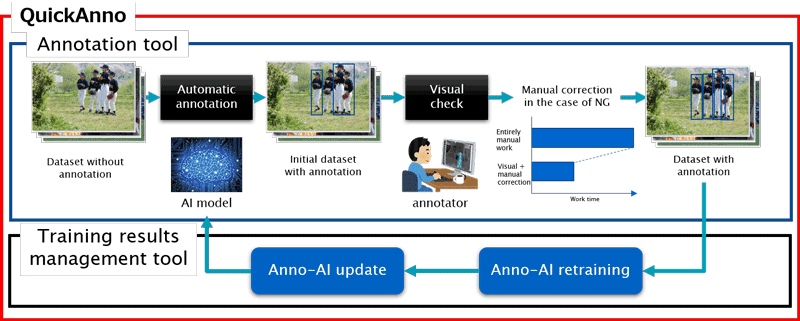
Fig. 2 Workflow of Annotation in QuickAnno
In addition, the linkage with MLflow enables centralized management of the retraining results and model versions, sharing with other projects, and rollback to previous versions. Automatic annotation can be started efficiently even for new projects by using the past training results and similar models as the base. All these operations can be performed through the integrated GUI on a web browser (Fig. 3), ensuring ease of use without expertise. The current Anno-AI model lineup provides a speech-to-text model in addition to models for imaging tasks of object detection, classification, and segmentation. Konica Minolta will continue to expand the lineup.
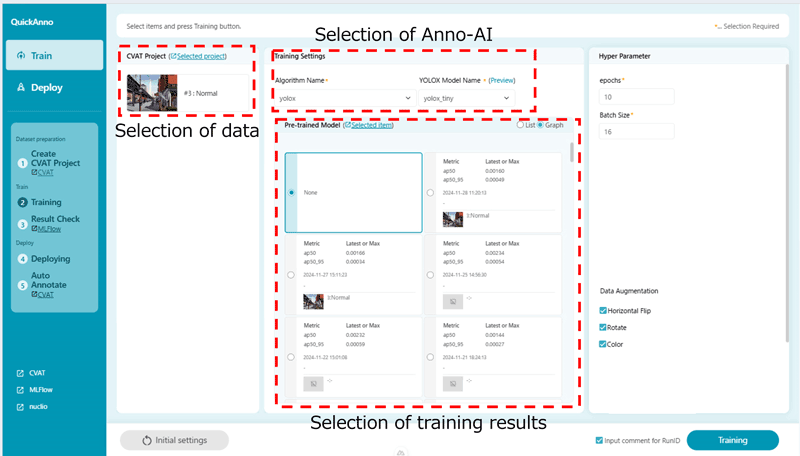
Fig. 3 Training GUI
■Future outlook
This system cannot fully demonstrate the accuracy of Anno-AI easily when the number of datasets is small. To overcome this difficulty, an AI model that maintains stable accuracy even with a small amount of datasets will be added to Anno-AI to realize high-accuracy automatic annotation from the initial phase and further improve the versatility and accuracy of this system.