Materials informatics (MI)/process informatics (PI)
Innovation of materials development techniques and production control technologies by informatics
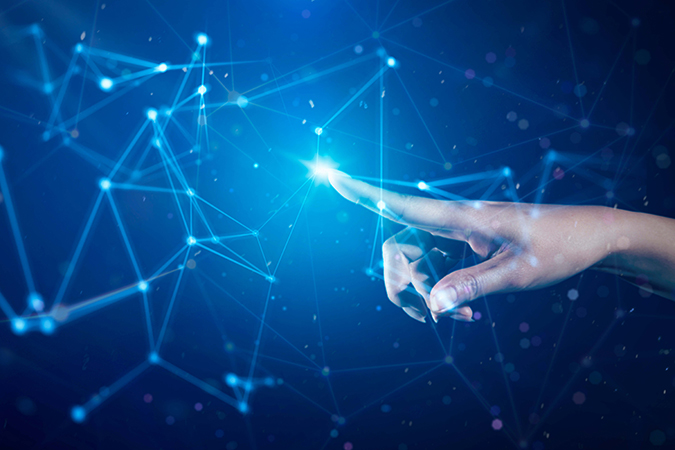
Core technologies combined with informatics
Konica Minolta promotes smart manufacturing by combining informatics with its existing core technologies. The company uses materials informatics to create high-value-added products and facilitates on-demand manufacturing to create customer value. Also applies process informatics to improve the efficiency of production and the entire supply chain in order to use energy and resources more efficiently.
Technology Overview
For its MI and PI, Konica Minolta uses diverse informatics techniques, including simulation techniques (first principles calculations, molecular dynamics calculations, finite element method, etc.) and imaging-IoT technology which combines sensing and AI technologies (rule-based systems, machine learning, and deep learning) and other techniques. By combining these technologies and materials science, Konica Minolta aims to establish innovative material development techniques and production control technology.
The company is also developing and introducing cutting-edge AI and simulation technologies and actively collaborating with external research organizations, including participating in national projects.
Materials Informatics (MI)
While polymer composite systems are considered difficult to analyze by MI, explanatory variables have been completely described for all experimental formulas by devising a mathematical way of expressing materials [use of a descriptor which is a combination of 0 and 1 for each brand of component materials (polypropylene resins, fillers, and additives)]. Using these described explanatory variables, we built a predictive model of elastic modulus by means of regression and successfully reduced the residual error to 300 MPa or less for all data points but one in experimental validation. This level of residual error was very close to ε2 (309 MPa) in leave-one-out cross-validation (LOOCV), demonstrating the high accuracy of the constructed predictive model.
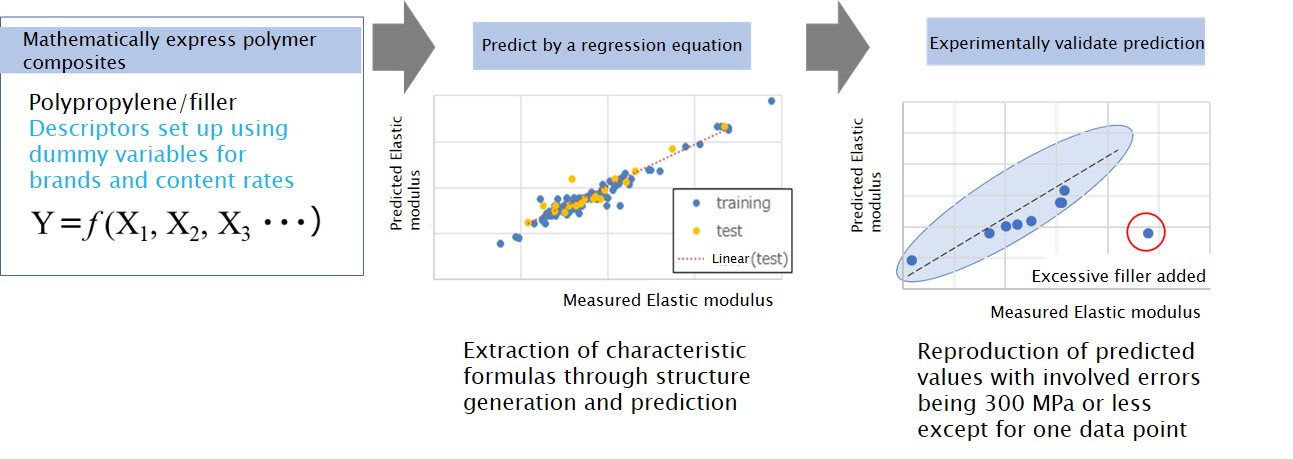